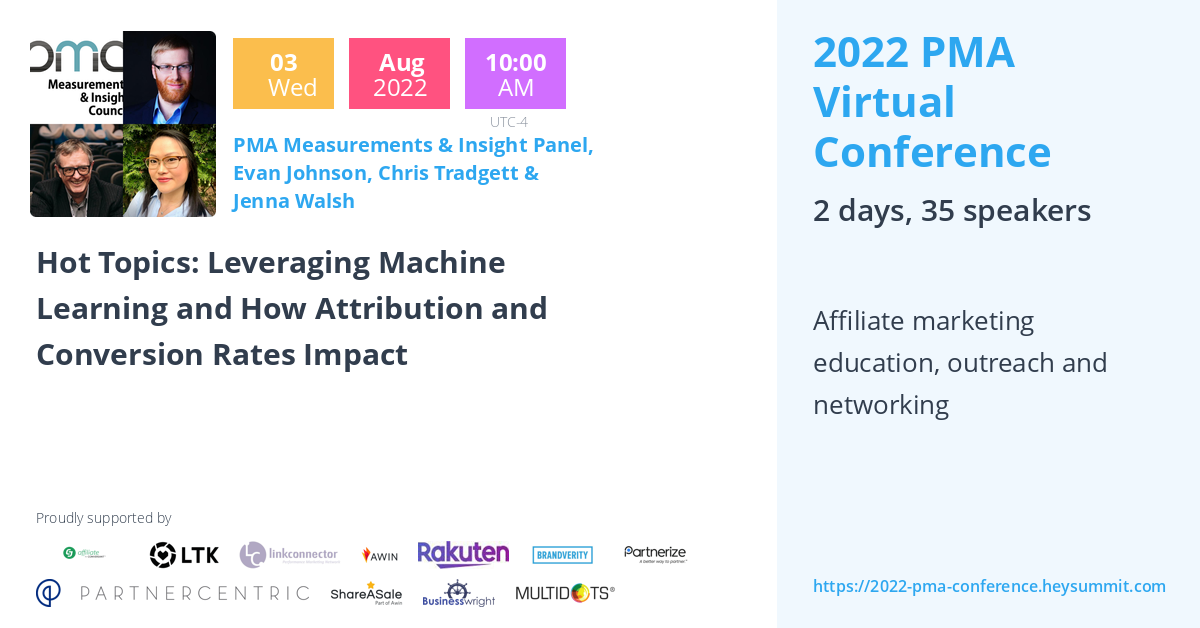
The Measurements and Insights Council Talks Hot Topics in Data: Machine Learning and Attribution
Contributors:
When we think about how affiliate marketing has evolved over the years, data is at the center of the conversation. As a follow up to our virtual conference, the Measurements and Insights Council wanted to recap our discussion around Machine Learning and Attribution, as well as add some key takeaways.
To kick off our conversation, we discussed what machine learning is and the different types of machine learning models: supervised learning (finding a trend line that best fits the data) and unsupervised learning (cluster the data into groups). According to Wikipedia, machine learning can be defined as a field of inquiry devoted to understanding and building methods that ‘learn’, meaning methods that leverage data to improve performance, make predictions and carry out certain tasks*.
So how do we leverage machine learning with an affiliate program analysis? We discussed a few examples:
Publisher recommendations can be far more valuable with the use of machine learning, and we see Networks utilizing this today. In order to effectively recruit and add new publishers, you may use the traditional KPIs and answer the question: Who performs well for other programs within my sector but not in my program? But, you may want to filter and ultimately shorten the list of recommended publishers based on particular models such as semantic search or clustering. You may also want to take into consideration KPIs which you didn’t initially consider such as average days from activation to click or average days from click to sale. Adding a machine learning value includes the predicted performance, but also keeps in mind the speed of which performance will likely occur.
We can also leverage machine learning to detect atypical program activity and mitigate against tracking issues. For a simple approach, we could create a benchmark based on consistent program and sector trends, and flag anything that would be an outlier. Ultimately the goal here is to inform, but machine learning can be used here also to predict future program discrepancies.
Another way that affiliate networks can use machine learning is with predictive analytics. One case study we looked at asked the question “how can we better predict the sales of particular publishers” and asked that question to a series of neural networks. As with any neural network, the more data it is fed the better, so make sure if you are undertaking a similar project to identify, gather, clean, and process the data most relevant to your question. Our case also showed the importance of testing several different models and checking their accuracy with the real results, whether that be over the short term or the long term. After training these neural networks and comparing them to the actual results, we are able to use a model which can accurately predict the sales that publishers will bring. This is critical whenever a network is working with future predictions, ranging from making forecasts to win new business or predicting current business to maximize financial liquidity efficiency.
AI and Machine Learning is being applied to affiliate recruitment with a number of new tools available, such as Publisher Discovery and Grovia from Acceleration Partners. In this example report from Publisher Discovery we see that keyword search enables an affiliate manager to search outside their network. Here we see a search in the ‘Entertainments’ vertical, to discover affiliates with the specific keyword of ‘streaming’.
That enables the user to see all the networks and programs being promoted by that affiliate across any affiliate network and see how relevant they are to recruit.
It’s also possible to search by merchant program to conduct gap analysis and recruit their competitors’ affiliates; for instance Bloomingdales can easily see which websites are promoting Macy’s or other competitors. Machine learning gives an understanding of their potential.
Machine learning is also being applied in analysis of affiliate tracking and the effect of cookie compliance platforms. We have all long heard said that 5% – 10% of links don’t track properly and the new technology from Moonpull is enabling networks and publishers to discover where these dysfunctionalities are occurring.
Machine learning helps in the understanding of how the tracking is working throughout the handover from the affiliate website to advertiser – as well as on subsequent navigation to ensure that tracking is still occurring.
The analysis and reporting enables a joined up technical conversation between all parties involved, to ensure that issues are quickly resolved. More importantly, the links track and commission and override is recorded, which is in everyone’s interests in the affiliate relationship.
In addition to machine learning, we also chatted a bit about attribution and how this has been a hot topic in data, specifically in affiliate for quite some time now. We featured a case study from SingleView featuring Honey, where a client wanted to understand the true value of working with Honey and the best way to utilize Honey’s strengths. The data approach was to benchmark Honey’s performance against the wider affiliate channel and the client’s site itself. Over the course of the test, AOV stood out amongst the KPIs as a success. Honey’s AOV was 9% higher than the site’s average initially and by the end of the test the AOV increased to 13% above average. In addition to the strong AOV, Honey’s solo and first click revenue also increased from 4% to 38%.
Key Takeaways
Working with data is nothing new to digital marketers, and it is exciting to see how different actors in the affiliate space can leverage new tools, particularly machine learning, in order to get better answers to their questions. As the industry as a whole grows, the variables affecting our businesses will only increase in complexity. This can be daunting for companies always trying to maximize efficiency and incrementality, but fortunately as the demands increase so do our capabilities. We highly recommend companies to closely analyze their own data and implement tools such as machine learning, not just to maximize return but to give everyone involved better understanding.